Introducing Kaptain
Kaptain, our enterprise MLOps solution, helps you answer the question: how do I get a return on my AI investments? With a familiar environment for development in Kubeflow, plus all other technologies needed to deploy and scale models in production, you can get results faster with Kaptain. And safely as well: we address all critical CVEs in our components.
Kaptain is seamlessly integrated, tested, and deployed via helm in multiple different environments: public clouds, on-premise, edge, hybrid or multi-cluster with EKS and AKS, and air-gapped environments. Additionally, Kaptain is rigorously tested on Nvidia GPUs and Nvidia DGX boxes.
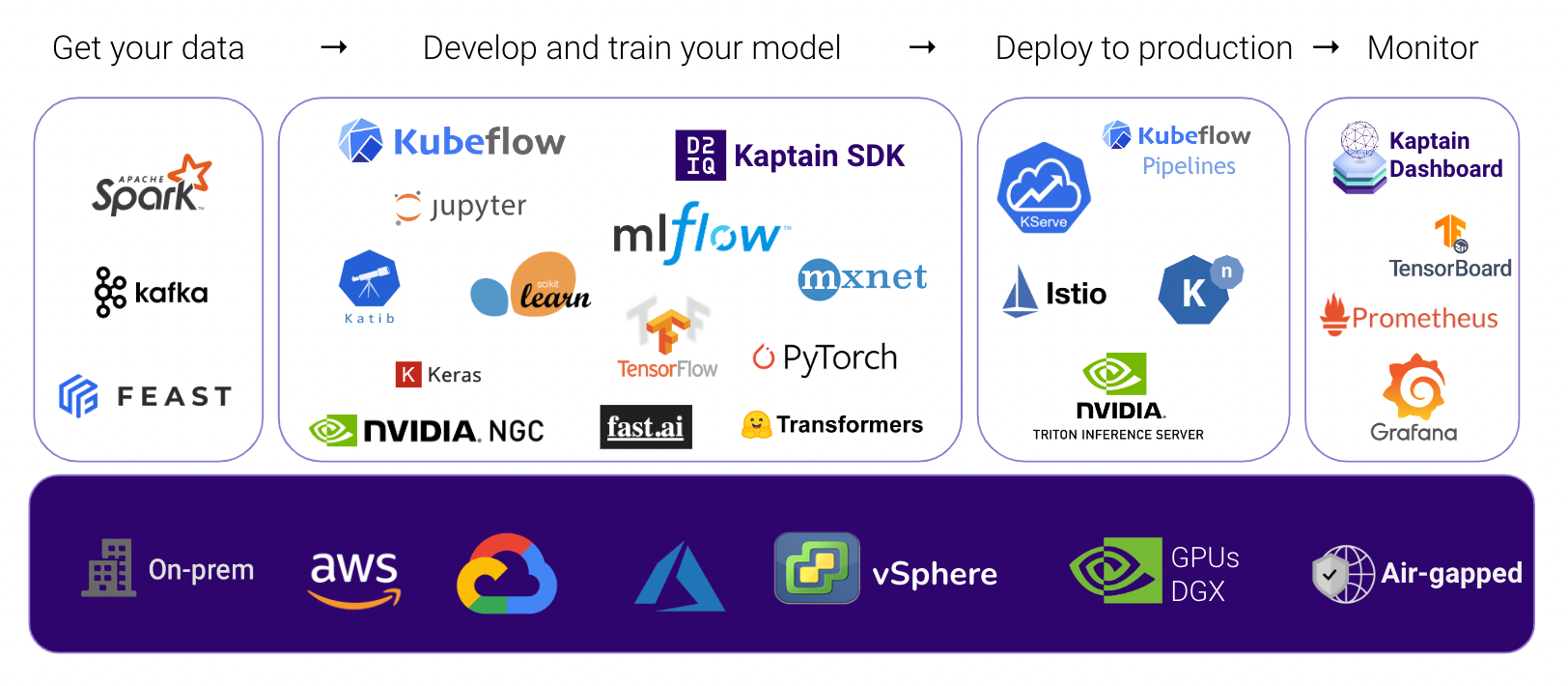
Kaptain’s Features and Benefits
Features | Benefits |
---|---|
Out-of-the-box integration of Spark | No need to install additional libraries to create data pipelines or train Spark ML models on multiple CPUs or GPUs |
Fully tested pre-baked notebook images | A familiar environment that has been fully tested and integrates with all the shared resources (CPUs, GPUs) and data access controls needed to build and share models as a team |
Train, tune, and deploy from a Jupyter notebook | No context switching or credentials and CLI tools on individuals’ laptops |
Enterprise-grade security controls, profiles, and identity provider support | Multi-tenancy? No problem! |
MLflow experiment tracking | Utilize MLflow’s robust metadata tracking within Kubeflow to get the most out of your experiments |
Garbage collection | Configure automatic cleanup of completed and idle workloads created by Kaptain components or the Kaptain SDK |